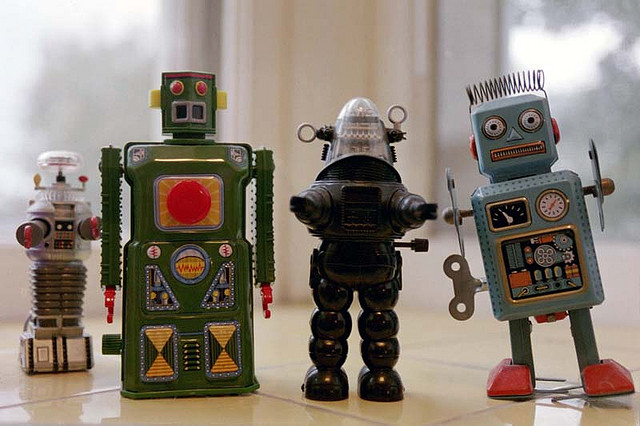
Automation still has shortcomings. Photo credit: Pyri Herrera
Automated sentiment analysis uses software to analyze the tone of content – usually media mentions about organizations, brands or issues. The software decides if a media or social media mention is positive, neutral or negative.
The software is used mostly by companies that offer media analysis and measurement services to client organizations. (Another application: Stock market analysts use sentiment analysis software to determine investor sentiment about the market and specific stocks.)
The software programs can shift through massive amounts of content quickly. Large companies, those with more than 15,000 or 20,000 mentions a month, like the time and cost savings the automated engines provide. In recent years, natural language processing has helped improve their analysis. The engines are consistent and are not influenced by biases like human reviewers may be.
Although the accuracy of automated sentiment analysis has improved markedly in recent years, the various software products still don’t match the precision of a human reviewer who is well-trained and experienced in media analysis. The automated sentiment engines tend to stumble over nuances of human language. Sarcasm and slang, which evolves quickly on social media, is particularly challenging. Plus, syntax on social media is different from the spoken word and normal written communication.
Estimates of items improperly classified range from 33 to 80 percent, according to Michael Blowers, owner of Media Evaluation Research in the U.K. He noticed that sentiment scoring of social media mentions of cigarettes seems to be remarkably poor. The same tweet frequently received a different score when retweeted. About a week later, analysis of mentions on mobile phones has much improved.
Not all sentiment engines are equal, Blowers warns. Sentiment engines that have undergone some kind of sector-specific training seem to be more accurate. Client companies rarely have a choice of which sentiment analysis software to use; it is selected by the media analysis and measurement service – and is the same for all clients.
The Human Advantage
While not perfect in their sentiment assessments, human media analysts are trained to recognize language nuances and idiosyncrasies that the software-based systems miss. Humans are also less literal than machines and can better tease out meaning. Native language speakers living in the country of publication are best at making those types of judgments and inferences.
In addition, a single version of sentiment analysis software may not be equally effective for different companies. Sentiment analysis can differ from industry to industry and company to company. Example: most sentiment analysis software typically accepts “fraud” as a negative term. There’s little question that “fraud” is negative if used in relation to a financial institution (unless the media clip discusses how the bank, insurance company, or brokerage is working to prevent fraud).But a company offering anti-fraud protection software may view term “fraud” as positive or neutral in most every case. Humans are able to differentiate word uses in context. Machines often cannot.
Fake robot accounts are another issue. Social media platforms are overrun with fraudulent accounts. Facebook alone estimates that it has 83 million fake accounts. Any automated analysis will surely evaluate mentions produced by fake accounts – and skew sentiment results. Humans are better able to detect and discount fake posts.
Howard University marketing professor Angela Hausman, PhD, takes a dim view of sentiment analysis. Excited by the lure of “big data,” many firms believe only an automated tool can analyze the vast amount of data available. Why, she poses, should they analyze so much data, when the inaccuracies are extensive and costs of training a tool are ongoing? A better option would be to manually analyze a random sample.
Companies using software-mediated sentiment analysis are best served by having human reviewers double-check results. That, regrettably, requires added time and expense. But it’s clearly the best solution to assure the most accurate sentiment analysis and measurement results.
To optimize both results and expense, reviewers can check only positive and negative results. Checking at least a random sample is recommended. When large numbers are involved, a selective random sample can be just as accurate as analyzing all clips. A random sample, however, may miss micro-trends.
Bottom Line: While automated sentiment analysis is improving, accuracy levels are still less than desirable. The best option for large organizations with large quantities of media mentions is to combine automated analysis with human review. For smaller organizations with a limited amount of media exposure, human review alone is usually the more accurate and cost-effective approach.
William J. Comcowich founded and served as CEO of CyberAlert LLC, the predecessor of Glean.info. He is currently serving as Interim CEO and member of the Board of Directors. Glean.info provides customized media monitoring, media measurement and analytics solutions across all types of traditional and social media.
Great post, when dealing with large amounts of data a combination of human and automated review is usually the most effective.